A new study from China has introduced an innovative solution to transform air transport in an era marked by rapid urban expansion and increasing traffic congestion. The research, focused on advanced air mobility (AAM), showcases a novel multi-agent reinforcement learning (MARL) approach to effectively manage the growing demand for air mobility, particularly in densely populated urban areas.
AAM is at the forefront of modern transportation solutions, aiming to improve the efficiency and environmental viability of urban airspace. Using new aircraft designs and cutting-edge flight technologies, AAM proposes a highly automated, safe and efficient method of transporting people and cargo through urban, suburban and rural areas at low altitudes.
Addressing the challenges of vehicle coordination in structured airspace
The latest study, conducted by a dedicated team at the University of Tennessee, Knoxville, addresses one of the most challenging aspects of AAM: vehicle coordination at critical merging points and intersections in structured airspace. The proposed MARL framework enables autonomous traffic management, ensuring safe and efficient navigation through these complex airspaces.
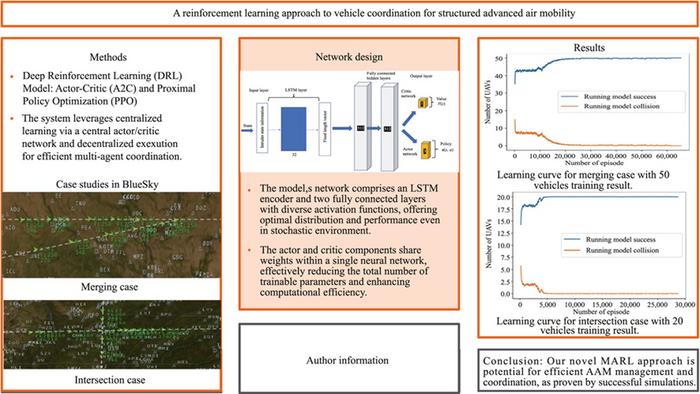
As urban areas face increasing air traffic, traditional air traffic control systems, which rely heavily on human controllers, are becoming inadequate. The research presents an intelligent system in which AAM vehicles are guided by sophisticated algorithms capable of making strategic decisions in real time. This system not only improves safety by preventing potential conflicts in the air, but also significantly reduces travel times and mitigates traffic congestion.
Deep reinforcement learning approach enables seamless vehicle coordination
The cornerstone of the study is its deep reinforcement learning approach, which allows each AAM vehicle to operate independently while seamlessly coordinating with others. Through extensive simulations using the BlueSky air traffic control simulation environment, the researchers demonstrated the effectiveness of their model. These simulations integrated real-world data to provide a robust evaluation, highlighting the system's ability to handle high-density traffic scenarios safely and efficiently.
Furthermore, the study reveals that the implementation of such advanced systems can lead to a significant change in the dynamics of urban transportation. By reducing reliance on ground traffic and promoting a shift to the skies, AAM offers a dual benefit: relieving traffic congestion at the city level and contributing to a reduction in urban air pollution.
“This research not only charts the course of future urban air mobility, but also establishes a benchmark for the integration of artificial intelligence in the management of complex transportation systems. As cities continue to grow and demand for efficient transportation solutions increases, insights from this study will be invaluable in guiding the development of sustainable, efficient and safe urban air mobility systems,” according to the researchers.
Leave feedback about this